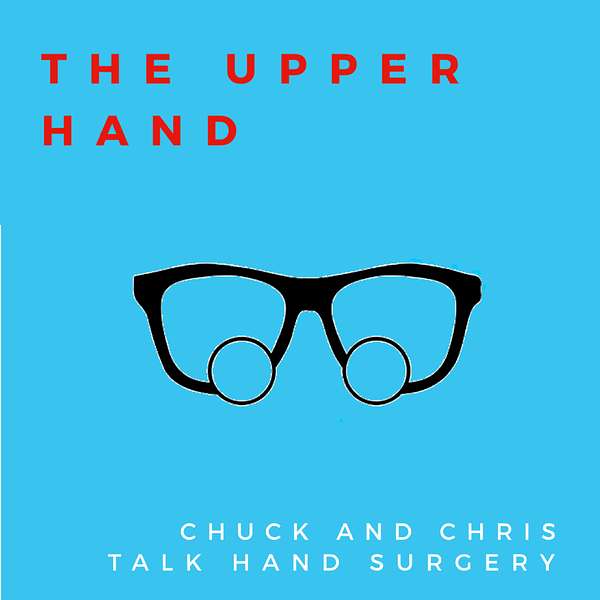
The Upper Hand: Chuck & Chris Talk Hand Surgery
The Upper Hand: Chuck & Chris Talk Hand Surgery
AI and Medicine: A Discussion with Alpesh Patel
Chuck and Alpesh Patel, Professor of Orthopedic Surgery and Neurosurgery at Northwestern, discuss artificial intelligence and its role in medical practice. In the first of a two- part discussion, Chuck and Alpesh start with the basics on what AI is and what it can reasonably do and they start to delve into more details around practical applications.
Subscribe to our newsletter: https://bit.ly/3iHGFpD
See www.practicelink.com/theupperhand for more information from our partner on job search and career opportunities.
The Upper Hand Podcast is sponsored by Checkpoint Surgical, a provider of innovative solutions for peripheral serve surgery. To learn more, visit https://checkpointsurgical.com/.
Please complete our Survey: bit.ly/3X0Gq89
As always, thanks to @iampetermartin for the amazing introduction and conclusion music.
Complete podcast catalog at theupperhandpodcast.wustl.edu.
Chuck, welcome to the upper hand podcast where Chuck and Chris talk hand surgery.
Chris Dy:We are two hand surgeons at Washington University in St Louis, here to talk about all things hand surgery related, from technical to personal.
Charles Goldfarb:Please subscribe wherever you get your podcasts
Chris Dy:Thank you in advance for leaving a review and a rating that helps us get the word out. You can email us at handpodcast@gmail.com so let's get to the episode. Hi there. Upper hand podcast listeners. This is Chris. We're back with an episode that's dropping a week early. Chuck is going to be interviewing Dr alpesh Patel from Northwestern University. He is a spine surgeon, but has expertise in lots of areas that are broadly applicable to all of our listeners, including artificial intelligence. So check it out. I'll be back for our end of the year episode that is going to release at the very end of December.
Charles Goldfarb:All right, I am here today with a really special guest. Chris Dy is not with us, so it's going to be myself and Alpesh Patel. Alpesh and I have known each other for a long time now. He was three years behind me finishing his residency at Wash U in 2004 prior to that, I think he did undergraduate at Cornell MD at Northwestern. There's a theme coming and I would love to talk about this, maybe another podcast. You got your MBA from Kellogg, which is super impressive, and you have been on faculty at Northwestern since you finished your fellowship. You are a well known and renowned spine surgeon focusing on the cervical spine as I understand it. And I love to talk anything businessy or life with you. And today we're going to talk about AI. So tell me what I missed. Tell me what you want to add. Yeah, welcome.
Alpesh Patel:Yeah. Thanks so much, Charles. I appreciate it. Are we going by Chuck or Charles? What's your official podcast name?
Charles Goldfarb:Yeah, you know, I wanted to switch to Charles as I got older, because I thought it sounds more, you know, adult. Yeah, I can't, I can't do it when I went to business school. I'm like, All right, I'm going to be Charles. I'm going to introduce myself as Charles. And I failed, so I'm trying, yeah,
Unknown:okay. I mean, you've been chuck to me for for, you know, 24 years. So I'm going to stick with that one. That's a hard habit to break, but, you know, thanks for the introduction. I do appreciate it. I will make one little mini shout out just in that, in that bios that I did spend five years, five wonderful years, at the beginning of my practice at the University of Utah. And so I was there, yeah, with, with Charlie Saltzman was my chair, and he had hired me out there, and I phenomenal mentors. It actually plays into a lot of my career trajectory as you'd imagine, right? A lot of things you see early in your career have this tendency to propagate and and really push you forward and propel you forward. So I had a five wonderful years there with with Charlie and Darryl Brock Hughes, the current chair. And then I came back to Chicago and and that is where I was born and raised, and outside of a period of time, in college, then training it in residency with you, and then those few years in Salt Lake, I've been in Chicago and affiliated with Northwestern for a large, a large chunk of that time. Yeah, no, I'm excited to talk. We talk about a lot of things, but I will, I promise you, I'll try to keep my answer short, and I will try to focus in on the AI conversation. And we've been doing some great work at Northwestern on AI machine learning and how, how it plays into the world of of patient care, specifically around musculoskeletal care. Yeah, perfect.
Charles Goldfarb:Briefly, before we jump into that, you are current President, I believe, of the cervical spine Research Society. And your meeting is coming up this week. I believe is that right?
Unknown:Yeah, yeah, absolutely. I think we are. I am the president of the cervical spine Research Society. We are a international organization focused on improving the care and outcomes of patients with cervical spine diseases through research and education. And we have our annual meeting actually in Chicago, just about a mile from where we're talking right now, and it should be fantastic. And a big component of that meeting, in addition to the human factor of getting people into a room together to exchange ideas, is that is a conversation around around research, and a large chunk of the research that we be, we see being submitted to the CSRS, to other societies, to journals, you know, is involves utilizing different types of AI based methodologies, right? And so, so I thought I appreciate you letting me plug the meeting, and I appreciate the chance to talk about it, but it does overlap quite a bit. Yeah, I think you probably see that in hand. We see that in a lot of surgical subspecialties as well, right? Yeah,
Charles Goldfarb:for sure, it's interesting. And we're gonna, well, I'm gonna, I'm not gonna say, I was going to say that it's interesting how research is now far more than just about the nuts and bolts of a surgery or taking care of a patient. There's so much else that's brought into the discussion of research. It's all relevant. But you know, when we started in residency. There was none of this. It was just the nuts. And
Chris Dy:please make sure to appreciate our sponsors. The upper hand sponsor@practicelink.com the most widely used physician job search and career advancement resource. Becoming a physician is hard. Finding the right job doesn't have to be join practice link for free today@www.practicelink.com you
Charles Goldfarb:All right, so let's jump into the meat of the conversation. And I first heard you talk about artificial intelligence at an AOA meeting, American orthopedic Association meeting. It's probably been five years ago. I don't remember exactly when it was, but it was an excellent I think it was a symposium. It was excellent symposium, and got my wheels turning. I hadn't thought about it a lot before. Then I've thought about it a lot since. So let's start with some definitions, easy ones. What's AI?
Unknown:Yeah, so that's these are good places to start, by the way, right? Because I think many people listening, myself included, have heard these words thrown around for a long time. I mean, AI, we I heard, I heard about artificial intelligence back in the 80s, when I was just learning about what computer science was and what what you know, basics of research were. I think the best way to think of AI is to think of it as a broad field of computer science, right? So it is a large field of computer science that attempts to create systems and processes that mimic, or, I should say, really recreate tasks that human intelligence would normally have required to do. It is, it is, I think the key differentiator here is that it's, we're not talking about a general AI that mimics a human being. I think that's where science fiction takes us. That's where all of our books and movies and have taken us, certainly out of my childhood was watching those movies growing up, so we need to erase that from our conversation a bit. We're not talking about a general sentient AI. We're talking about tasks, tools, processes that we can think of as taking on the tasks that would normally require human intelligence. Perfect.
Charles Goldfarb:And how do you think about the definition, the excellent definition you just gave it AI versus a definition of machine learning.
Unknown:Yeah, that's a good one, because you oftentimes again hear these used together and used interchangeably. So I would again machine learning. Think of it as a subset, right? If AI is this large circle a machine learning is a smaller subset of that. It's an example of artificial intelligence. So think of it as a subset of AI that involves basically teaching computers to learn through different techniques, using different algorithms. And what that learning means can vary from technique to technique. Some of it's what's called supervised learning, where there's a human teaching and algorithm, there's unsupervised learning, where you have the algorithm sort of teaching itself through iterative learning, and then you've got other examples of that that blend the two right between supervised and unsupervised. Sorry about that. I'm getting a text from my residents in the morning. Hopefully that doesn't pop up on your screen. So so that's where I think of ml. And machine learning comes in lots of different ways and forms. It has lots of different goals, but generally speaking of it, I would have most people when we introduce them to the concept thinking of it as an example of artificial intelligence. It's probably the one that we have investigated the most, because it's mostly algorithmic software driven, and the availability of those software is pretty widely spent right now it's pretty widely available. So the limitation there really isn't the access to data. Some of the other applications of AI require, I think, a lot more upfront technology. And that's where you won't see it, maybe as widely applied, yet it's sitting mostly in the hands of private companies or, you know, large collaborations. So
Charles Goldfarb:the machine learning that affects you and I so if I go on to one of the, you know, chat, GPT or Claude or whatever, and type in, you know, what is carpal tunnel syndrome, the machine learning that we get there is that harvested from web crawlers, basically harvesting as much information as possible from as many sites as possible across the internet. Is that where that information is gleaned?
Unknown:Yeah, so, so chat GPT is one that we all have heard about, right like, and I think it's pretty common. We certainly I have it on my phone and I think, and I use it probably on a daily basis at this point. Do
Charles Goldfarb:you pay for it? Do you have the $20 a month one we pay
Unknown:for I pay for it because it's we also utilizing this part of our research, levels of depth of it that we need, that we couldn't get through it. But for a long time, outside of that, for day to day life, I haven't paid for it. You know, the searches I'm searching up don't require the speed that we're talking about for subscription service, nor do they require the complexity, you know, we I used it to come up with names for our turkey trot team. You know, that offloaded some work from my from my head, you know, about a month ago. But also for real things around work as well. But chatgpt, again, it's an example of AI. It utilizes machine learning methodologies, right? But it's not machine learning by itself. There's a there's a large language model behind it. There is some predictive analytics that go into it. There's iterative learning as well. It also does allow you to use voice, right? So there's a, there's a sound component to it. You can upload images to to it now, so it actually has computer vision baked into it, which is another subtype of AI, right? So, So machine learning is one component of it. Think I would think of it almost and this is overly simplistic, and more savvy listeners will challenge me on this, but I think of machine learning as the some of the gears that go in behind the machinery, if you will. If I use a 18th or 19th century analogy, but, but that's a but chat GP is an example of where we see a potential impact of AI applications in to us as physicians, to us as patients, right? We're all patients at one point in another, but even to us as individuals, maybe running businesses or running research efforts, is that this is a, this is the first large scale, highly visible Well, talked about, you know, AI application that that we've experienced in our lifetime.
Charles Goldfarb:Love it. So one of the things our listeners are thinking about now, if they're thinking anything like I'm thinking, is, you know, and we're going to this is going to go in all different directions, because it's very hard to have a straight line discussion about this. But you may have answered one of my questions, which is, which engine I guess is the right expression do you use? And it sounds like you use chat GPT. I've done a lot of kind of trying to investigate, and actually in business school, we talked about this. You know, there are a number of alternatives by your choice. I'm assuming, did you prefer chat GPT over Claude, over Google, Gemini over perplexity, over Microsoft, copilot, et cetera, et cetera? Did you choose chat CBT because it's better, or did you choose it because it's it is what it is? I don't know. Why'd you choose? Yeah,
Unknown:yeah, let's be clear on that one, by no means am I advocating for one machine over the other. It was the first one available. It was readily available. So, right? Go you learn in business school, right? Go to market. If you got first mover advantage, right? You got a big advantage. And I think chat GPT lives that it's got first mover advantage, it's got brand recognition, right? So when you think about these AI based let's call them assistance for right now, right? Or AI based platforms, it's the one that everybody's heard about. So that's why we use it. I use you mentioned a couple of the other ones. Gemini, I've used that on my phone. Co pilot, we have that available as well. Which one do I go to? You know, again, I wish I could say that's my day to day life, that that's driven by a lot of in depth thought analysis and pros and cons. It's really like, well, chat. GPT is the first one that comes to top of mind for me. Now, what I would say is, from a research standpoint and from a clinical application standpoint, it's really important conversation to have right which is to say, can we rely on one platform or one engine more than others? And the absolute answer in 2024 as we speak now, going into 2025 is that we cannot, I don't think anybody could say that one of these platforms is better than others. When we look at it from a research standpoint, we have to really ask hard questions, which then which then inform our clinical applications. Is, are these really reliable, right? Right. Chat. GPT is sort of a generalized, large language model. It may not current state be trained on In Depth medical content, for example, let alone spine surgery content or hand surgery content or the very, very small details that go into our world. There's also, sorry, may not have the depth of knowledge, because it doesn't have the data in, because it does grab data from. I mean, where they get their data from is proprietary. You don't really know for sure, and as the lawsuits come out over the years, we'll find out where the data comes from. But the thought process is that the data was captured or through large public, publicly available data sources, right? Um. Um, but when we think about which of these is best, you're what you're seeing right now in the market is that you'll see, let's call them AI companies, because that's what they'll market themselves as. But application companies that are solving for very unique issues, right? They are going to come out and solve for a very specific problem, whether that be a specific diagnosis in our language as physicians, a specific procedural solution, a specific thing, they're going to be hyper specialized, AI platforms or systems, rather not not generalized platforms, because they're limited on their data that puts in and AI ml, what we've seen from our work at Northwestern is that it's your data in drives the quality of the outputs, right? If your inputs are marginal or questionable or inconsistent, your outputs will be so there as well.
Charles Goldfarb:Love that. I'm going to again, I'm zagging a little, but touching on something you said a year ago, because things are moving very, very quickly, we heard a lot about one of the limitations you mentioned. One of the limitations being access to knowledge and in depth information and things like carpal tunnel syndrome. One of the other limitations was the risk of incorrect information provided with a search and that some would label hallucinations, so I might type in what's the best way to treat carpal tunnel, and they may the response may be rotator cuff repair or something just completely bizarre. What's your sense of the state of the quality of the information that we receive back from a query? Yeah,
Unknown:I think it's, again, it depends on the question that's being asked. If it's a very specialized or specific question, I think you still need to be very skeptical of the answer. If it's a general question, you may find that the answers are fairly accurate and fairly reliable, and you may find that the more you ask it, the better it the better it understands what you're looking for, right? So a lot of the suddenness comes down to the prompts, the asks that we put out there to these systems. And we have to get better at asking better questions, maybe. But some of it is also the actual data in and the example I'll share is we have a research project right now that we're that we're submitting, so that this is not peer reviewed yet. This is just going into into the peer review process, but where we compared, for example, three or four different engines looking at looking to do a systematic literature review, right? So you remember Chuck, when we were residents, we would do these systematic reviews. Our job would be to scour the literature and then look for every peer reviewed journal we could find, look at all the references in those peer reviewed journals, and keep digging and digging and digging and
Charles Goldfarb:walk to the library to do that. Yeah,
Unknown:I'm just trying not to date ourselves that much. I do think we had a computer to search on, but nonetheless, you would do that iterative process, right? So if you think about a human task that might be replicated by an algorithm, by machine learning algorithm, part of an AI platform. Yeah, that seems like a repetitive human task. So let's see. Let's compare. So we actually compared our residents doing a literature, a systematic review on a specific topic in cervical arthroplasty and cervical fusion surgery. And we compared that to three or four different models, one of them being chat GPT, so the other three being more specific sort of medicine or healthcare specific models. And what we found was that there was a fair amount of overlap that that we found a lot of the articles that the humans found, which was cool. We like that's always feels good. There were some articles that our residents found that the mission that the algorithms couldn't find, but then there were articles that the algorithms found, and chatgpt in particular, found that not only we couldn't find, they actually don't exist, right? So we identified a number of articles that were suggested to be actual review articles actually not review but primary sources of information to include in a systematic review that actually don't exist. They've never been published. There's no authors of that name, and there's no papers of that name. So that's an example where an output in a hyper specialized question you have to be very skeptical of, right, very skeptical of, but when I asked chatgpt for, you know, a recipe for a really good, you know, old fashioned, it does a pretty good job of coming up with a good recipe for an old fashioned. So I think you have to, we as orthopedic surgeons, we as researchers, need to temper our expectations from these products for now, that's not run towards some finish line. And then we also need to be really good advocates for our patients, right? As always, we want to educate and advocate our patients, and they may find themselves with these technologies in hand, expecting them to be sources of truth, right? And so we need to be able to explain to them. Be, not just what a reality might be, but why they may not be able to put all their faith in that, in that platform. Yet,
Charles Goldfarb:med students on the back, I did, I think my second AI sort of related research. And Carrie Reaver, who's now a second year med student, did an amazing job. It's been accepted for publication in jdjs, which is awesome, looking at the readability of online patient education materials in English and Spanish, assessing how chat GPT did and reading level and all that stuff. So there's so many directions. This an example of paper that wouldn't have been published when we were residents. But there's so many different important things to do and different directions to go using chat GPT, so you're doing really cool stuff. This is a little bit of low hanging fruit, but I think important. No,
Unknown:I think it's really important. And I think that's an example right there, Chuck, and we've looked at a similar thing, which is to say, Hey, listen, you know, we've invested a lot of time and effort into this patient education, content, materials, is it readable? Is it understandable? And we're finding again, and this is where a machine learning program has helped us get through that process faster of understanding it same things, right? It gives you a lot of insights. So there's that's an example of where we can take these tools and actually use them for a very specific question, a very specific purpose, a very specific output, and rely on them, right? Yeah, so they're hyper specialized tools, yeah, and that's accessible when we trade treat them as general tools, we start to see limitations fast.
Chris Dy:Mark your calendars for March, 7 and eighth, for checkpoint, surgicals. Next category course, restoring hand and wrist function, optimizing surgical results and avoiding complications. Join the course faculty, dial Kyle Dr Kyle chapla, Dr Amber lease and Dr Deanna Mercer in Las Vegas as they review management strategies to assess, preserve and restore hand and wrist function. To learn more about this and other educational programs, please visit nerve master.com checkpoint driving innovation in nerve surgery.
Charles Goldfarb:One important thing to mention for all the listeners is, you know, how do you handle HIPAA and important patient related information. So I'll tell you what we do. I'd love to know what you do. So we have very specific guidelines at Washington University that I think are pretty well thought out. So we I cannot take patient information or Excel spreadsheet and use chat GPT as publicly available, but we have a protected playground environment for it's called WashU chat GPT, and in that environment, I can do whatever I want, because it doesn't go out, it only comes in so that I can do and can't do. We're on zoom right now, the Zoom AI tool we can use, the Adobe AI tool we cannot use, and that's been turned turned off. What other examples, or how do you think about that? Or did I capture a good amount of it? No, I
Unknown:think that's a really good example. I think everybody you know, whether you're you're sitting where we are, which is inside of a university system, right, which has a lot of processes in place for for data protection and data privacy, patient privacy. Sometimes I find myself trying things that wanting to try things and I'm told we can't do that. And while I'm frustrated at as a, as a, as a researcher, I also understand why we're why we have these constraints, right? Because there is a massive privacy concern that comes with it. If I'm in a in a smaller group practice, let's say as an orthopedic surgeon, right? Or or in a in a private company or small company, this is something that you really need to spend time thinking about, which is, what are your security measures going to be? And I think you're finding, obviously AI companies that are solving for that. That's the beautiful thing about capital markets. If there's a problem, you're going to generally find a solution. But yeah, we have the similar kind of constraints. Northwestern, for example, has a has a strategic partnership with Microsoft, and so that gives us some degree of freedom to work within a constrained firewall, if you will, for lack of a better term around copilot, right? And when we work with other algorithms, what we're doing is making sure that all that work stays internal, that we're not actually actively sharing any of that information externally. That's something that again, 10 years ago, when we started this work on predictive modeling, that was never a conversation, right? And so now it, thankfully, is a big part of the conversation, one
Charles Goldfarb:of my it's not a, not a fun example, but just how careful we have to be. There was a plastic surgery group in town that was posting pictures with, you know, patient privacy pictures, but they were unlabeled. Patients were really hard to identify, but there was metadata. Uh, associated with the pictures with patient names that led to a massive lawsuit. The message being, we have to be really careful here.
Unknown:Yeah, no, for sure. And again, we there's a lot of these anecdotes, right? There was a story about about or there was an example of a group that took just to demonstrate the privacy risk that we may not be thinking of, that took LinkedIn images that physicians had posted on LinkedIn that had any kind of an x ray or a CT scan of the head and neck, and they were able to take that data and using an AI algorithm, you're scaring me, recreate faces of the people who were supposedly, you know, de identified, anonymized, and they could recreate faces of who they think these people might be. And and now you may argue, well, they aren't accurate. They can't really do it. But the idea is, you know, we're not that far away. Then, if it's, if the first go is not that is an idea in that direction. You'll iterate, and you'll see people figure it out. So even things that we think are anonymized, we really need to ask, you know, twice and ask thrice and really find, like you said, firewalls that protect our ability to try things out from, you know, unintended consequences. Absolutely,
Charles Goldfarb:I want to ask you one question, which I think you could probably talk about forever, but I'm going to ask you to keep it brief, because I want to get to the medical applications of AI. When you, let's say you're doing a search, and you're on chat, GPT, and you, you know your query is, what's the best way to treat cervical pain, I don't know. Something explain to the to the listener that hope I'm saying this correctly, that this is not a one question, ask chat GP and walk away with the answer. Explain how you question and then refine your interaction with chat GPT to get to the answer you need.
Unknown:Yeah, no, that's a good point. So so from a Research Lens, we oftentimes ask one off questions, because that's the research methodology, right? And so you you have to think about it in the real world application. It's not a one time game, it's a repetitive interaction. So again, we we when we look at how these models function through their interaction with us as end users, as humans, right? And this speaks to a bigger issue, which is that, you know, in current state, ai, ai applications are really meant to augment us as people, not to replace Right, right? So if they're augmenting us, they're helping us with knowledge, decision making, insights, predictive modeling, whatever it might be, but they're augmenting us. So it's our interaction with these platforms is really important. So what's the iterative prompt? Right? How do you get really good at prompting the different platforms to get you the knowledge that you want to get to at some point, not to get too meta here, at some point, there'll be an AI platform that does the prompts for you, that will iterate your prompts for you as it gets to know what you're interested in, right? So that you can ask a relatively simple question, and the AI will figure out what you're really asking. But we're a ways from that, right? We're away from that, probably in a good way, that we have some barriers there. So it is a matter of asking again, I think the take home is, if you want to interact with one of these platforms, ask a very specific question, know what you're looking for? You yourself should know what is it that I'm really trying to figure out, does surgery help cervical radiculopathy? Right? And if so, how often? How frequently, what can predict a successful outcome versus not a successful outcome? Now, I would argue these are questions right now that as a patient, I hope they feel comfortable asking me, but in that, you know, compressed time that we have with patients in the office, we may not be able to get to the depth of this, of the question we the patients, may not feel at ease oftentimes asking these kinds of very, very specific, data driven questions, or they may not even know what questions to ask, right? So that's where an assistant like this can give a patient information. If I'm being very optimistic here, information, at least with directionality, right? We may not tell them the final answer, but it gives them an idea of what direction should I head and what kinds of questions should I ask, what kinds of things should I be looking for? And that's how I try to educate my patients on how to interact with
Charles Goldfarb:with these platforms. Yeah, it's we hear a lot about the number, or the percentage of patients that walk into the office have been having interacted with the Internet. And by Internet, traditionally, we talk about Dr Google, and I think in St Louis, it still is Google, but that is going to change pretty rapidly, I think, to interactions that are with chat, GPT or others. So even
Unknown:right now, Chuck, if you go to Google today, the first thing that. Pops up as a Gemini answer. That's exactly so even if it is Dr Google, it's still going to be a lot of it might be informed by that top line that people see right which is, which is, this is Gemini answer? Yeah,
Charles Goldfarb:that's a that's a great point. All right, let's get to the meat of this discussion. And and I knew this was going to be a time challenge, and I know we talked about keeping this to 45 minutes, we'll see this is great stuff. So if you want to
Unknown:do a part two, we can always do a part two on the on like, the in depth applications, right? Because that's, I think a lot of times, what people are wondering is, what's out there right now, and do I need to incorporate this into my clinical practice? Do I what is going to look like in 510, years, right? Like,
Charles Goldfarb:yeah, that's actually a good I'll
Unknown:let you lead the store. I'll let you lead the way. Yeah,
Charles Goldfarb:we'll see. We'll see what we can get accomplished and what we feel like we left on the table. So I'll start with a broad question, and then we should obviously take it to a different level. Tell me how you and how Northwestern is incorporating AI on a clinical and educational perspective, maybe not so much the Research Lens, but clinical and educational
Unknown:perfect. Yeah, no, I'll, and I'll speak mostly for myself. I will say what we think what we're doing within orthopedic surgery and spine surgery at Northwestern I'll give some examples of what we're doing at a hospital level. But it is, I would caveat and tell everybody this is still relatively nascent, right? Like we we are we in academics and in large healthcare systems are usually not first to market, to move towards things, right? So we're taking this a bit slower than you might see in the private world, right, in non healthcare applications. So how do we use this? So there's a couple of things. If we think about clinical work and we think about who is a technology going to benefit? Is it going to benefit a patient, or is it going to benefit us? Maybe like as this physician, surgeon, healthcare team, right? So, if we think about it as us, as a as surgeons, I think many of us, many people listening, may already be utilizing some degree of an AI based technology without necessarily overtly thinking of it as AI, right? So one example would be, is if you do any kind of preoperative planning, and if you use any kind of software for pre operative planning, which in the spine world is again, still relatively nascent, but we're moving in that direction, right? We work with one company that that uses AI technology to design custom made patient implants for spinal surgery that help us, help guide us towards or lead us towards more reliable, consistent outcomes, right surgical outcomes in terms of spinal realignment. That's just one example. That is an AI based technology that is already in in in real life, already being utilized, yeah, maybe at scale, because there's some cost issues there still, and we still need long term outcomes to know if we're making an improvement. But that's one example, which is like pre op planning we think about, you know, another example for patient facing lens, you know, is around education. We talked about this earlier, right? Can we do things to make patient education simpler and more reliable? And so one thing that we've tried it is research. We got to see if we can roll this out, is to, is to take this one problem, which is MRI reports, right? I'm not sure if you have this in your practice. I know I have it in mind. Many of our partners do, which is that our patients will will nowadays have ready, ready access to all of their health care information. Wonderful. I mean, the that level of transparency is fantastic, but all the information in there is not at a level of readability that most people, even the most well trained, well educated person, may not be able to understand it, and they'll come in with their MRI report in hand, a highlighter in the other hand, and then just highlights everywhere. So we're taking these large language models and a couple different algorithms and trying to convert that language into something that an average person in the United States, whether that be fifth grade or eighth grade reading levels, can understand? Yeah, right. That's a patient based application that we'd love to see if we can roll out awesome.
Charles Goldfarb:Those are two good examples. Let me ask you some more specific ones. Absolutely. So when you see a patient in clinic, do you dictate your note? Do you type your note? Are you using an AI assistant to create your note. What are you doing now and then, I'll tell you what I'm doing. Yeah.
Unknown:So what I do is I dictate a note using an application. That application has in since the time we started with, it been bought and sold and bought and sold and is now sits inside of Microsoft, and they take that recording. And it used to be that that recording was sent to a person to transcribe, and I'm fairly certain now that that recording is transcribed by it by an AI algorithm. So we get it back, we are trialing a and. Be it listening technology, right? That listens to the conversation and creates a structured note out of it. We're in a trial mode there. That's again, another partnership with Microsoft. What do you do? Chuck? What do you do in your practice? If
Charles Goldfarb:I'm reading between the lines, and I never shy away from, you know, getting to the kind of specifics sounds like you're trialing DAX co pilot. Maybe, or maybe you're not. But we have, we did a trial using DAX cope out. And for those who aren't aware, Dax is basically Dragon, which was nuanced, which was purchased by Microsoft, and I have to say, when I trialed it nine months ago, it didn't, didn't meet, didn't meet our needs, and so now we are trialing a bridge technology. And just for those who aren't aware, I may have mentioned on the pod, I'm not sure, but basically, what happens and a bridge is great. We're moving on to the second phase, and we're going to start purchasing licenses. But it's another cost center, another overhead generator. But what the way a bridge works, which is the same way docs copilot, works, is I have to open my epic application on my phone. I put the phone in the office. It listens, it records, and usually within one minute, I have a note which has been generated. So it takes a conversation and it reorders it, and it's super impressive. I would say the HPI and the assessment and plan are 95% amazing. The physical exam really depends on me awkwardly verbalizing what I'm doing, and it's, it's super awkward. So a bridge for me has been a game changer.
Unknown:Yeah, I think, I think that's a great example chuck of how, not only do our technologies iterate, but we need to be able to iterate right in our practices. We need to be able to be critical and say, Hey, you're there. You're not there yet. I think that. But this, that's just an example of, gosh. I mean, I will tell you like, I don't know a single physician who loves documentation. I can't think of a single person who says I can't wait to go out and document today, right? And we know the implications, like, we know the downsides, right, that this has had on the profession in terms of be
Charles Goldfarb:specific there for so, yeah, what are the downsides that burden?
Unknown:Yeah. I mean the burden, it's not so much the idea of documenting. We've been documenting for generations. We need that. That's how you remember what happened in the past. That's how you guide the future. You take implicit ideas in your head and you make it explicit in writing so that you you can remember and then others can pick up for you, right? If you're not there, there's a fundamental purpose of documentation. What's happened, though, right, in the last 15 to 20 years is really that the purpose of documentation has pivoted, right? And it's no longer about patient care. It's about it's about coding, right? And it's about coding to the maximum amount you can while also meeting all the regulatory requirements. So you layer in those factors, and now documentation goes from a useful tool to a burden, and that burden gets magnified. How many patients a year do you see? Chuck?
Charles Goldfarb:I see 60 patients a clinic times, you know, 50 weeks a year, and occasionally some other clinics thrown in. So, yeah, that's 3000 but it used to be four to five, but I've cut that well, yeah, you're
Unknown:more important now, so you can. So I'm right there. I probably see about three to 4000 people. We've got some of our partners that are in sports medicine that are seeing 8000 9000 people a year. And then we think about our primary care docs who see maybe, they may maybe have a panel of 2000 or 3000 but they're seeing them so many times, that's a ton of work, and that is time and effort that takes away from us talking to patients. And I think beyond the fact that it just sucks to sit at a computer, is the idea that we're actively being robbed of time that we could be spending with a patient. So this is a in its create. We think it's a driver for a lot of physician if you like the word burnout, or don't like the word burnout, or if you think it's an injury or not an injury, but a lot of the dissatisfaction of being a doctor, oftentimes, it pretty consistently, comes back down to this documentation issue. So if you can take an AI solution and make that more you know, make it simpler, make it more reliable, right? And make it somewhat automated so it's happening in the background that should make things better. I I'm trying to imagine, in my skeptics mind, how does that make things worse, other than an accuracy issue? What is it that would what that would create in terms of a negative and it's really net positive?
Charles Goldfarb:Yeah, I think that's all really well said. I do think I'm gonna add add to what you said. You can correct me if you can correct me if you don't agree, because I'm not trying to inappropriate. So first of all, you know, yes, when we dictate our notes, it's all about coding. It's also about cover your ass and medical legal. And it's always been that, but with electronic medical records, you know, they don't have to interpret my handwriting to assess, you know, what I may have said. So, and that's good. And the second thing I'll say. Is I do. It's very clear that the documentation load, especially for those in primary care specialties, Does, does increase the dissatisfaction with career choice and the word burnout, as you said, I would say my experience with these clinic dictation assistants is incredibly positive it. And I find that, you know, what we all don't like is if we go to, if I go to my primary care that I really like, I'm sitting here and he's sitting here typing on his computer, and that's miserable. No one likes that with this tool. You know, I'm having a great direct conversation with the patient not writing anything, and so the connection with the patient is markedly improved. And the price of this technology is not a huge burden, but it comes with at least the way we're thinking about it is, if I'm going to pay, and I don't, you know, some doctors have scribes, and scribes are very expensive tools. This is far less expensive, but it's still another cost, and I better see one or two more patients in clinic to cover that cost. And that's just what medicine does. Every layer we add brings an expectation we need to generate more revenue. Is that off base? Or what do you think?
Unknown:I think I listen, I totally, I totally agree with you. I learned when I was your junior resident to always agree with you, right? So what I would add to that, though, is it's, it's, yes, you want to be able to offset the cost, right, that you're adding to your practice. There's another component, which is, which, when you're running a business, right, and you're running a group, which is the idea of, like, Can you, can you hang on to talent, right? So you may say, Listen, yeah, this is an additional cost. And if I ledger it and I line item it, how do I balance it out? Is this going to make my physicians, my surgeons, my nurses, my mas pas pts? Is it going to make them more likely to leave me or less likely to leave me? Yeah, right. So talent retention, acquisition and retention, I think, needs to be added to that mix that you just threw in there, which is, hey, listen, it may not give me that time to see an extra patient or two, but gosh, you know what it does? It makes my makes my my partners really happy. It makes my nurses really happy. It makes my PTS really happy. And so we're going to make this as an investment, because it helps us for retention. That's just another example, right, of where you might find these tech, the application of these technologies beneficial to your to your clinical practices. And we see this being being mirrored in non healthcare industries, right? Chuck you. And I talked before about the benefit of getting an MBA is, hey, you get a chance to see what life looks like outside of healthcare. And you see this applied pretty widely already, right, in a lot of different fields, especially in retail, consumer goods, those kinds of things and and the workforce component is a large part of that, right? It's a large part of their decision making is not just, can I get more productivity, or can I reduce labor, it's also, can I hang on to talent?
Charles Goldfarb:Really, really good and important point. All right, I'm going to put you on the spot and maybe make you feel uncomfortable. And I'll start by saying, I have done this. Have you ever seen a patient walked out of the room being a little uncertain about what the diagnosis of the most appropriate management might be, and gone to chat GPT and said, Help me with this. I have a patient with X, Y and Z. What do you think?
Unknown:So I have I haven't yet. I'll be honest with you, I have sat there and I've scratched my head and I've wondered, would this be a useful application of chat GPT? But then I go back to the research we've done, and I and I say, Listen, I can't rely on it to make a decision. So what do I do? I fall back right now into old habits, which is, I go back and I look at the literature, I do a PubMed search, and I look for relevant articles. Now that's time intensive. And so yes, would I eventually love to automate that? I would love to, but I've got to really come to a level of trust right on, on the sources of data that I'm, that I'm that I'm using, whether it's directly myself or indirectly through a platform, and I'm I'm just not there yet.
Charles Goldfarb:Yeah, I would say this, that's a really important point for all the listeners. Is, you know, maintain a healthy skepticism for me and my little world of congenital anomalies and syndromes. If I have an undiagnosed patient, putting a constellation of phenotypes or appearance issues can be helpful, but that's more, you know, add add up a number of factors and help me understand so very different than a true clinical intervention scenario.
Unknown:So Chuck, I would actually, can I ask you a question? I know that I'm your guest and you're the host, but so would you have you ever taken, uh, or thought about taking an image of a hand anomaly and putting that out there, just the image, putting that out to the world and saying to the to a platform and saying, What is this anomaly? And can you detect it through through a computer vision application? Yeah, it's
Charles Goldfarb:a great question. We have not done that. We have talked about that as a next research step, because it is a great question, because I'll give an example. I had an amazing young family come in an office last week with a child with a congenital difference. And you know what we do now is we, you know, you and I, and anyone who's seeing a lot of patients, no matter what the potential diagnoses are, we're just sort of doing pattern recognition. Well, pattern recognition is exactly what what this is, and I didn't know what the diagnosis was. So in our research group, you know, we have the code registry, which is really an amazing platform, and so if we don't know the diagnosis, we check, we check a box, and our classification committee considers it. But your point is excellent.
Unknown:That's a great structure, by the way, to have in place, and I would commend you to be willing to put it out there to the world that sometimes you know, and you're one of the you're not going to say this, but you're one of the nation's experts in congenital hand differences, right, in anomalies. And for you to say, Yeah, we may not know sometimes, and I'm willing to check a box that says I don't really know that, by the way, itself just speaks to like a learning environment, a growth mindset, all these super positive things. I also will say, I appreciate you use the word difference as opposed to congenital hand defect, which I think is what it was called, right when we were in training. So I think that's a great application of it. So I haven't used it what I have done, though, with patients, because sometimes we're having a conversation and and even if I'm not dealing with the level of Rarity or uniqueness that you are, there are times where I will be talking to a patient about a condition, and it may be a cervical radiculopathy, a lumbar radiculopathy, it may be cervical myelopathy. So really common conditions for us as spine surgeons, but obviously brand new to the patient. And I will just ask them. I said, Hey, can I have your phone real quick? Let me type in the condition so that you can start to figure out what to read about on your way home or when you get home. I'm not sure if our phones are actively listening to us or not. I'm, you know, my, my they are, as a lay person, I think they are but, but I can't count on that, so I give them, I'd sit there and I'll type it in for them, right? Whether it be into it's usually Google. And so these are the things I want you to read about, or I will specifically send them to, you know, surgical videos that I that I vetted already. So that's the the depth of Interplay right now that I am comfortable with, with patients. In general, I have had patients of mine that are coming, whether it be from Northwestern or from one of our other teaching institutions in Chicago who come from a computer science background, and then it's a very different conversation, right? Because they're interested in this. I'm interested in this, but I try not to, I try to have a mental barrier up where I'm trying not to let it influence my my decision making, because I just don't know if I can rely on it or not, right? But you said a couple things. Pattern Recognition like these are broad concepts, but what is a what does an algorithm do that we're already doing? How does a computer take what's implicit in our head and make it explicit? Right? To the world? Pattern Recognition is one you do predictive modeling in your clinic all the time. Chuck, right? You're looking at a patient, you're looking at their diagnosis, you're looking at the structure of their hand, you're looking at the parents, you're looking at the kid, and you are putting all those factors into play to try to predict an outcome. That's right, and that's essentially what a machine learning program is trying to do. If you if you do a predictive modeling program, it's taking data out there and try to predict an output. The one thing that it can do differently is that it may find factors that we can't even identify. Right and that's the cool part, but the hard part, and this is actually what led us to that AOA symposium, to go full circle, was that we don't necessarily how do we interpret the output if we don't understand what the inputs are in a machine learning model, and if it's truly a black box, if machine learning, and all of these applications to us are a black box, and we all we see is the output, but we don't know what's going on in the inside. We don't know how the sausage is made. That's a problem, because then we can't be critical of it. And I think that's what led us to the AOA symposium, was we thought, you know, listen, this is growing in our research. It's growing in our clinical applications. Most orthopedic surgeons were not raised in this world and don't have the level of critique, or, you know, critical thought, critical tools, I should say, to apply here. And can we move the needle there? Right? We know how to, how to, how to look at a chi squared analysis. We know how to look for a regression, a basic linear regression model. We know what the pros and cons are there, because we've seen that over and over again. We need to get to that same level of of comfort with some of the some of the AI processes. Yeah. Yeah, I
Charles Goldfarb:love that. I'll tell you where my brain went, and then I'm going to ask you to sort of close this down by listing a few other things either you guys are doing or thinking about doing. And maybe that's a teaser for a follow up, if you're willing, where my brain went during your conversation. Was I imagine this, you know, University of Chicago or Northwestern based computer science expert coming in your office with, you know, cervical radiculopathy, and you sit down and you're like, geeking out on this with this person, and all of a sudden, your clinic is totally off the rails. 30 minutes later, you're still in the room, and you're like, my day is shot. But you're super having fun talking about this stuff, because, you know, clinics go off the rails in so many different ways, but a great conversation with the patient. Sometimes it makes it okay, by the way, I
Unknown:think it also it always makes it okay. Now, if you're my next patient, you would not agree with that. That's right, but if you're my current patient, I think you do, right? And it may not be just a computer science issue. It may be more a matter of, like, listen, as we know, we had some great mentors, right? Like, and so we got to see if your patient feels heard. I think the number one complaint we hear from a patient, and in my practice, in many people's practices, that they The doctor didn't listen. No one listened to me, right? And that might be because we're click clacking away on a keyboard the whole time. It might be because we're already thinking about the 30 minutes I'm behind, the 45 minutes I'm behind. But if you have a way to connect to somebody, and if it's through something that you have a passion in Yes, I do find myself stuck in those conversations. I can usually tell them I'm in a rabbit hole, because the resident that I'm with or the fellow that I'm with, you can see them take their phone out slowly and start to be looking at their phone a little bit. But then I again, I also have a great team. And my team, you know, fights hard to keep me, against me, to keep me on time.
Charles Goldfarb:The funny anecdote, and I think the week, this has been said on the podcast that you and I both learned from my current partners, Marty Boyer, that, you know, 90% of the time the time the patient walks in the room, and within 30 seconds, within 10 seconds, we know the diagnosis. And so Marty will talk to the patient about their pot roast for five minutes, and that's the connection point. And we laughed at him in his notes talking about the pot roast, but of course, he got it right. I mean, he really has a way with most patients to connect in that regard,
Unknown:yeah, and this is a whole nother conversation, which is that that example you just shared, the ones that you probably have from your practice, and how you connect with the kids and their parents, right? The way we try to connect with our patients at Northwestern that is all about building trust, right for sure, and I will bring this full circle to the conversation around AI. So the issue here is, I think forever and a day we've believed, and we've lived in a healthcare world where people have trust, like they come to the door trusting us, right? I think that that level of trust is really in question right now, and I think some of that level of trust being questioned is justified, right? And some of it, some of it is probably a very emotional, driven questioning based on what's happened in the last couple of years, right? So, but that ability to create and generate trust is still something that we have as humans that I don't know that an AI algorithm or an AI application is going to be able to replicate or replace now that can be challenged for sure, right? Like, I trust my Google Maps to tell me how to get home, like to cut through Chicago traffic. What's the right way I have trust in Google Maps to do that. Sure, if I'm searching on Amazon for something for my kids for Christmas, I trust in Amazon will show me their algorithmic process will show me things that you know that 11 year old boys or 15 year old boys like, right? Hopefully it's all safe for work stuff on the 15 year old boy side. So, but I think healthcare is different, right? And I think this is the fundamental question when we think about long term application of AI technologies, when we're talking about patient facing, applications, back office, stuff, revenue, cycle, management, marketing, regulatory, contracts, finance, that, that we're going to get to very, very quickly if we're not already there in some practices. But patient facing ones have to begin and end with trust, and that's still where we come in. And hey, you know what? If that automated scribe you describe gives you five minutes in the room to talk about, you know, what a patient's going through, or to talk about their job or their interests, or whatever great because you're that's the win building trust. That's the win. That's where a a surgeon working or a physician working with an AI technology is a better option than a surgeon working by themselves or an AI technology working by itself. Yeah. Right? I think that's where we go. This idea of human machine intelligence being a combined effort, not not a not a competitive one. All
Charles Goldfarb:right, we really do need to close it down. So I love some of the back office stuff you mentioned. You know, the coding process which we have. We hire coders who are wonderful, but you can see the writings on the wall where the future lies there, tease us with a couple other things either you're doing or or might be doing in the future. Yeah.
Unknown:So, so on the back office side, we there's so much talk about, and that's, again, not surprisingly, where you see a lot of the early technology being applied already. Yeah, right. So, so, so we can talk about that anytime that that's probably more of a business school conversation, but hopefully a lot of the physicians listening care a lot about that as well. I would say, from the surgeon facing side, where our research is going is around trying to get to better predictions of outcomes, right? Can we? Can we utilize machine learning insights to get to better predictions so we can better inform our patients what to expect? Let's say, after surgery or after treatment, because then that might affect their decision making. Right? All this comes down to supporting really good decisions in healthcare. We want to think a little bit about patient education. I mentioned earlier one example of how we're trying to translate medical or radiology as a language into, you know, patient facing language, English at a right reading level. And then how do we utilize, use AI technologies to better communicate with patients broadly? I think these are some of the things that we're working on, from a research standpoint, that I'm super excited about. And I think about the next five, six years for our for our research efforts, that's the fun part. And then there's some really, really tangible applications to solve problems for patients.
Charles Goldfarb:Love that. Look, I've taken an hour of your time, and we talked for quite a while the other day in preparation for this. This is This is gold, and I think our listeners will agree. But yeah, I'd love to circle back and go into more depth. Tell me who your most important partner is with your research and moving this field forward.
Unknown:Yeah, so I'll name one person. Sreed divvy is one of my partners at Northwestern he joined us in 2020 he's leading our machine learning and orthopedic intelligence process moving forward. And he's fantastic. I'd love for you to meet with him and consider, you know, having him jump on he's really been the, been the human engine behind our machine learning work. And then, again, more broadly speaking, though, you know, we're, we are just a couple of researchers in a really large institution, and we're lucky to have really great colleagues and with a lot more depth of expertise and in computer science. And then the last group, I'll say is actually, is actually our students and our residents that are right. We have a and three has helped develop this. We have this immense pipeline of talent going all the way up to the undergrad population at Northwestern who are deep into computer science and data science, and, you know, I have to, whenever they talk, I'm so blown away by how bright these students are. I also need to stop and take notes so I go home and I figure out what they're talking about, but, but they've also really been an unbelievable source of of ideas and talent, you know, for us, love it. Love
Charles Goldfarb:it. All right. Thank you for your time. I look forward to continuing this conversation. Continuing this conversation, and good luck with your meeting this week.
Unknown:All right, thanks so much, Chuck, thanks everyone.
Charles Goldfarb:Hey, Chris, that was fun. Let's do it again real soon.
Chris Dy:Sounds good. Well, be sure to email us with topic suggestions and feedback. You can reach us at hand, podcast@gmail.com
Charles Goldfarb:and remember, please subscribe wherever you get your podcast
Chris Dy:and be sure to leave a review that helps us get the word out.
Charles Goldfarb:Special, thanks to Peter Martin for the amazing music. And
Chris Dy:remember, keep the upper hand come back next time you